Data Virtualization – Why is it a necessity for data-driven enterprises
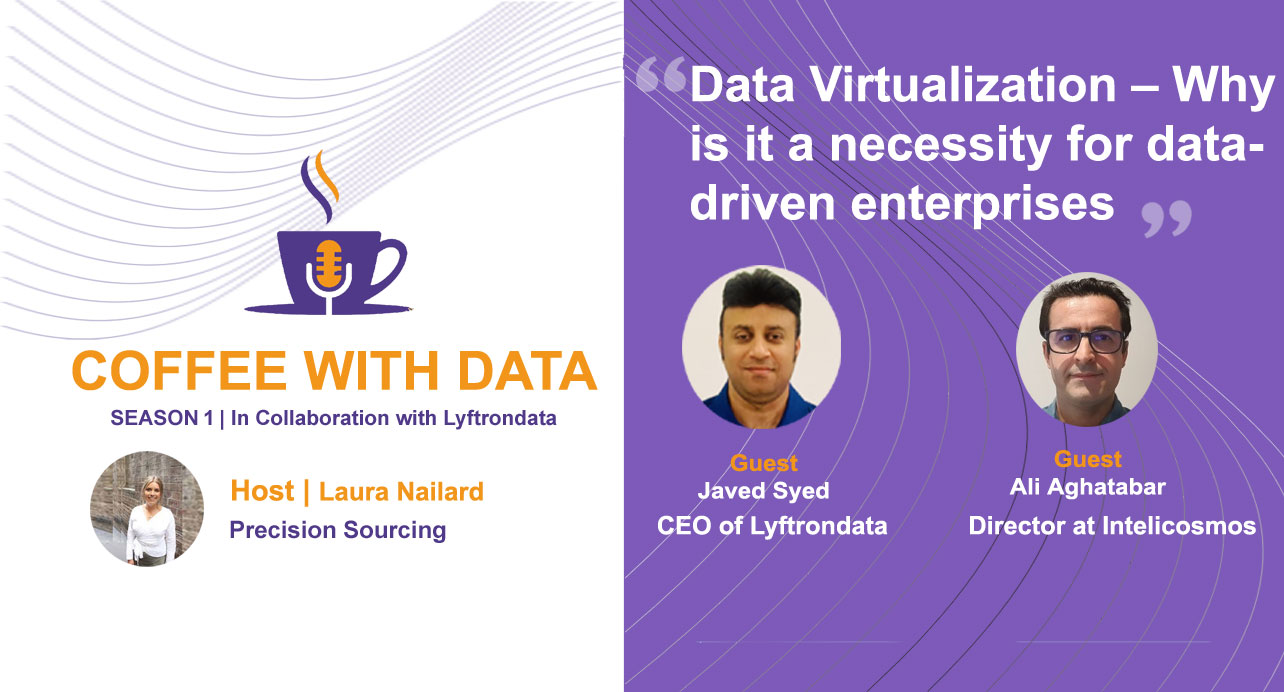
Join experts as they discussed what modern-day data virtualization is and how it has always been a crucial component of any data-driven enterprise.
Join experts as they discussed what modern-day data virtualization is and how it has always been a crucial component of any data-driven enterprise.
Data Virtualization is a broad spectrum that enables data management to retrieve and manipulate data without much prior knowledge of information technology. The technical knowledge like the process of data formatting from the source, finding the physical location of the database and enabling the customer to view the entire data.
Big data means a larger set of data full of complexity, regulated by traditional ways of data handling. Big Data is the combination of V3s i.e. Volume, variety, and velocity. It is a collection of structured, semi-structured, and unstructured data where data with numerous fields have varied statistical data whereas complex data may lead to finding errors in discovery rates.
Streaming Data is the real-time streaming of data in the continuous progression from different sources. It is a continuous flow of data generation without having access to all the data.
IoT means the Internet of Things. This enables the world to get connected with software, sensors, data analysis, global communication, etc. through technology. It makes exchanging information applications or software an easy task.
A Traditional Data Warehouse is an off-line on-site process of storing data locally in your office or another physical corporate warehouse. In traditional data warehousing hardware, technical assistance and manual running of data are required. It is also called an on-premises data warehouse.
Cloud Data Sources are normally used for loading data from some external sources into the system and this will enable the organization to the database for analysis. There are primarily three types of cloud data sources, which are relational, multidimensional data sources, and dimensionally modeled relational data sources.
The best-suited use cases and patterns for Data Virtualization are as follows:
In the Data Virtualization Deployment Model, the early implications of virtualization take place for deploying multiple server applications on the same system. The model includes fetching information, delivering training to the model, evaluating the performance of the model, and implementing deployment and monitoring.
Listen, Engage and Learn from Data Scientists and Big Data Thought Leaders.
The Coffee with Data podcast series is for data and business leaders to learn how they leverage the cloud to unite, share, and analyze data to drive business growth, fuel innovation, and disrupt their industries. The data topic covered shall empower our future guests and our engaging audiences – Data Governance, Data Management, Data Science, Data Quality, Data Strategy, Data Architecture, Data Analytics, Machine Learning, Artificial Intelligence, Data Security and Privacy, Master Data Management are to name a few.
REST vs GraphQL vs LyftQL APIs
Watch our most recent podcast and listen to industry experts as they discuss LyftQL...
Read moreJourney of the Data Warrior
A semi-retired promoter for the Snowflake data cloud and data vault, Kent Graziano...
Read moreData Pipeline Differentiator
In this episode, Bob Collie – Chief Technology Advisor at Zmclp...
Read moreHow to build data pipelines for Snowflake
Hear industry experts as they shared how data fabric platforms are empowering...
Read more